How can users be literate of systems that evade the comprehension of even the experts who created them? For generative A.I., such a literacy seems to depend upon terms of art: misleading homonyms like ‘explainable’, ‘memorization’, ‘natural’, etc. In the field of computer science, terms of art serve as metrics to quantitatively evaluate A.I. performance with the aim of achieving greater efficiency in the tasks those systems were programmed to perform in the first place. Apparently, machine intelligence has blasted off; it approaches infinity and beyond while we stand still.
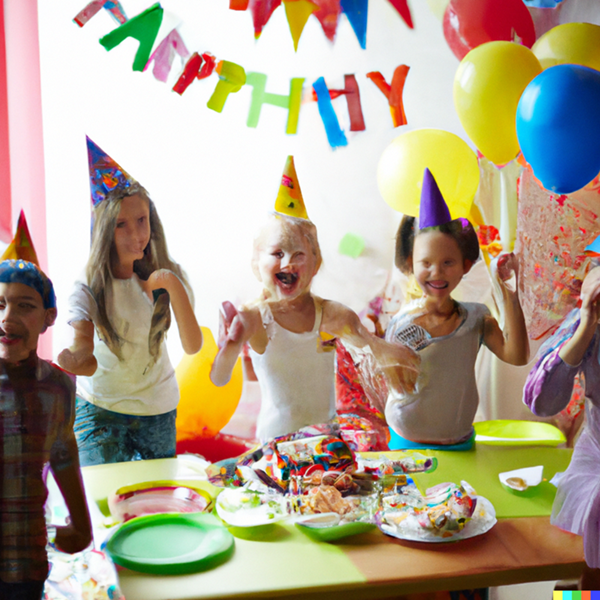
With this post, I attempt to carve off a clean slice of the on-going and unresolved research myself & my colleague Maria Baker have undertaken at Columbia University, working from our home-base: the Writing Center. Herein, I describe our method of reading across A.I. generated media in search of user-friendly A.I. literacy practices that counteract the shock and awe: The Layperson’s Pocket Guide to Resisting Rocket Science.
Counterintuitively, as teachers of writing, Maria and I’s research began by wondering if first looking at probabilistically-constructed images might help us better describe what we saw when looking second at probabilistically-constructed texts.
We began by reducing the complexity of our inputs to DALLE-2 with a variety of minimalist prompts like “birthday party.” The results of this auditing astonished us more than the wacky mash-ups, like “a polar bear playing bass,” which OPEN AI, the developer of DALL-E 2, strategically advertises as amazing and novel.
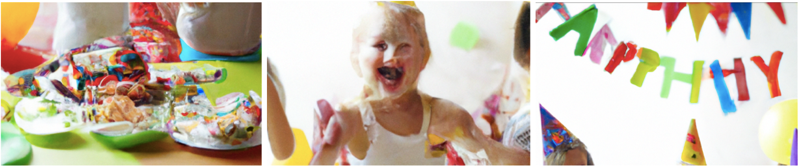
In the blink of an eye, hallmarks of machine assembly present themselves in this output. Overall, the incoherent seams of the pixel sequences turn the image eerie. The faces of the figures look roughly collaged: an absurd, open-mouthed, and frankensteined happiness. Very strikingly, the central figure’s face appears to be made out of icing. Objects like plates and snacks have no edges and spill into one another, ripped up like wrapping paper. The banner’s writing adheres to a plausible pixel-syntax, but doesn’t actually produce a recognizable word. Discordanly: the balloons are perfect.
However, this wasn’t what surprised us most.
We realized the color palette, the affect and attire of the figures, and the festive props all offer a familiar birthday composite, but one clearly adjacent to the staging of stock photos. With every prompting, DALL-E 2 would only generate such mangled meta-stock-images, compulsively adhering to what it ‘learned’ about birthdays.
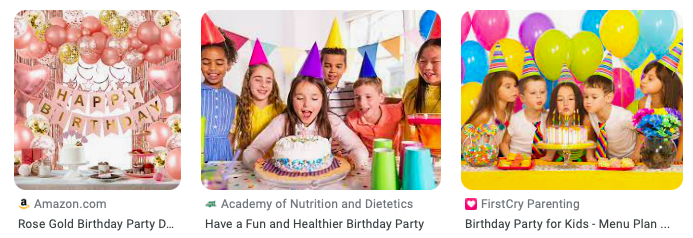
Over time, such eerie image outputs will become more ‘photorealistic’ (another utterly misleading term of art). Soon, every pixel sequence will appear as smooth as a party balloon. Regardless of this achievement, anyone investigating a dataset of “birthday party” outputs will still detect the durable and even eerier influence of stock.
For now, we merely note one simple consistency across all these birthday parties: everyone is happy.
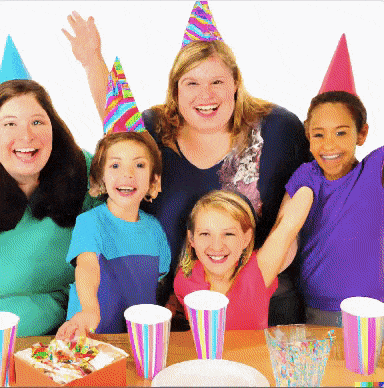
When compared to A.I. generated images of birthday parties, A.I. generated texts about birthday parties already appear flawless. Like the meta-stock-photo DALL-E 2’s image aspires to be, CHAT GPT offers meta-ideas for what a birthday party is supposed to be. What a birthday party is supposed to be, in turn, accords to the predictable ideas of birthday parties in stock photos. So this text passes muster. Unlike the A.I. image, however, it is the A.I. text’s very muster-passing-ness that makes it so inhuman, and presents the same eerie stock-dependence the image made so visible.
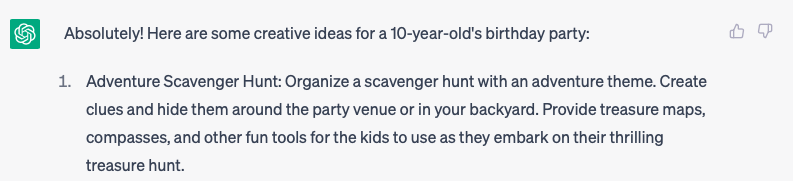
Who can find fault with this language? The text as text is unimpeachable (according to SWE). The output is also too likely to be wrong. No critique can be set against it. CHAT GPT’s birthday party meta-ideas were designed so that anyone could use them, and how could such generosity be a problem? Yet strangely, only certain kinds of families could inhabit these homogenized birthday party ideals (families with the “backyard” the bot presumes the user possesses). Despite the stock scenes the bot summons, some parents may struggle to replicate these parties IRL for their children, and instead notice how their families do not align with the norms machine intelligence presumes.
What goes up must come down. Our method allows a hard-to-name aspect of seemingly successful texts to be named. We name both image and text generated by A.I. as troublingly normative. DALL-E 2 or Chat GPT can’t know how narrow and particular their training data is. Machine intelligence has capabilities, but those capabilities end in the incapacity to know anything at all, especially any sense of everything that is excluded from that ‘intelligence’. Therefore, literate A.I. users would acknowledge what must be a corollary to that machine intelligence: machine ignorance.
Maria and I seek to cultivate in our writing students the very thing A.I. doesn’t currently, and we bet can’t ever, possess: high-quality ignorance. This kind of ignorance drives knowledge-creation by positioning inquiry at the threshold of what is already known, reflecting on the uses and limits of that existing knowledge. Our writing program borrows this framing from Stuart Firestein (a neuroscientist, ps).
Admittedly, to question the premises and promises of these A.I. systems will be hard. Especially for text generators: normativity will present itself in a form that defies easy perception. No reader will have a visceral reaction to CHAT GPT’s birthday party suggestions in the way a viewer might shrink from the inhuman renderings in DALL-E 2’s earliest “birthday party” images. Unless, like us, you can observe, in A.I.’s low-quality ignorance, a programmed certainty and a probabilistic guarantee that b-days are just plain peachy. Wouldn’t it be nice if the wish-fulfillment of machine intelligence were true: that birthdays are always happy and parties only fun; that parents always only love and provide for children who accept and celebrate themselves?
To be A.I. literate, our writing students (and developers alike) must question generative A.I. outputs critically. To this end, one feature of the technology is an incidental power to reveal its own limitations, even as it appears to race towards perfection at increasingly complex tasks. Acknowledging machine ignorance involves observing, naming, and describing elements of generative A.I.’s troublingly normative intelligence, which will be in effect, somehow, as a matter of principle and regardless of any given prompt’s sophistication nor any given output’s apparent mastery.
By acknowledging machine ignorance and questioning “what is compulsively included and excluded”, ‘users’ will transcend their status as such, and begin the demand for an ethical comprehension, beyond metrics, of the systems whose inevitable ubiquity only appears to be out of a layperson’s control.
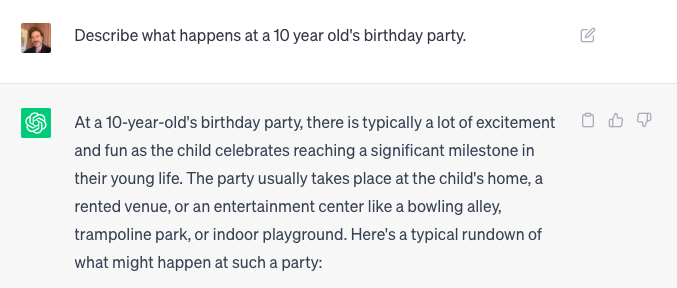